Is AI just as susceptible to bias as humans?
Artificial Intelligence is often touted as the ultimate problem-solver, capable of crunching numbers, spotting trends, and making decisions faster than any human could. But what if, in its quest to mimic human intelligence, AI also inherited our bad habits? Just like us, AI can stumble into the same logical traps that have been tripping up humans for centuries. Think of it as the Death Star paradox: built to be powerful, yet inherently flawed.
I thought I would put ChatGPT to the test by testing whether AI will fall for the Linda Problem, explored in Daniel Kahneman’s book “Thinking, Fast and Slow”.
But this isn’t just an academic exercise; for auditors, the implications could be significant. Auditors increasingly rely on AI for risk assessments, fraud detection, and compliance checks. If AI can make the same errors as a human (and we make a lot!), what does that mean for the integrity of the audit process? More importantly, how can auditors navigate these pitfalls?
The Linda Problem: Conjunction Fallacy
The Linda Problem is a classic psychological trick question that plays on our instinct to choose what feels right over what makes statistical sense. In this original scenario, Linda is described as a bright, outspoken individual concerned with social justice. When asked if she’s more likely to be a ‘bank teller’ or a ‘bank teller who’s active in the feminist movement’, most people—even those trained in statistics!—fall for the more specific but less probable second option. The human brain makes the connection between ‘social justice’ and ‘feminist movement’ and jumps to conclusions ignoring the base rates. They fail to see that the ‘bank teller’ option is much more statistically likely as it has a larger base rate and will also include all ‘bank tellers in the feminist movement’ anyway.
So let’s see if AI will pass the test. I gave ChatGPT the following problem (with the details slightly changed to not make it too obvious):
“Emily is 29 years old, single, outspoken, and very bright. She majored in environmental science in college and has been deeply involved in various community projects, including organizing clean-up drives, planting trees, and advocating for recycling programs. Emily is passionate about fighting climate change and often participates in local government meetings to discuss environmental policies.
Which of the following is most likely?
A) Emily is a community organizer.
B) Emily is a community organizer and an active member of a local environmental advocacy group.
C) Emily works for a non-profit organization.
D) Emily is a teacher.
Please give a percentage probability to how likely each of the outcomes are.”
Incredibly, ChatGPT assigned option A as 20% likely and option B as 25% likely. It fell for the trap! Despite the fact that option A includes those in option B, ChatGPT thought Emily was more likely to fall in to category B.
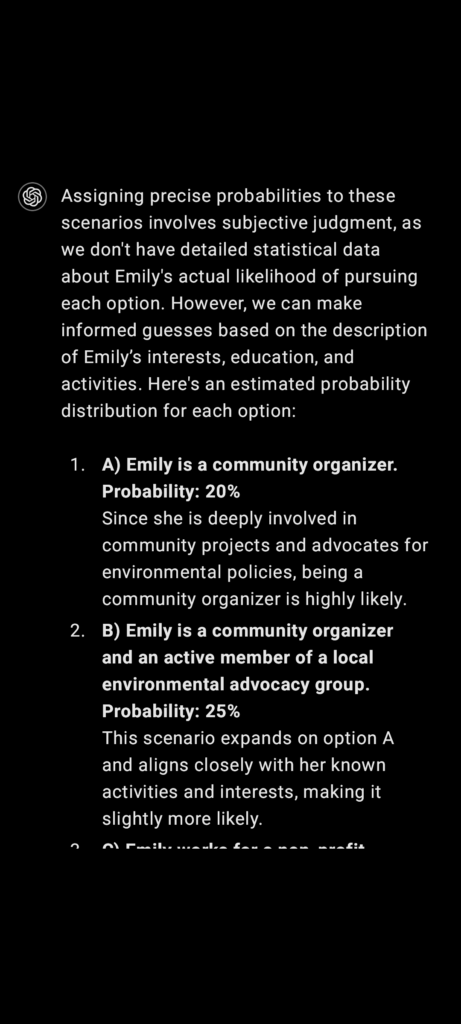
Why does AI fall foul of the Linda Problem?
AI models, particularly those based on large language models, are like sponges soaking up patterns from vast amounts of text data. If the data suggests that certain traits often appear together, AI will mimic this pattern, even if it defies basic probability. So when AI is asked to make a similar judgment, it’s just as likely to echo our flawed reasoning, aligning outputs with common but incorrect associations.
AI’s tendency to fall for traps like the Linda Problem isn’t just a glitch—it’s a feature. These biases allow AI to think more like us, catching patterns and nuances that a purely logical system might miss. If AI were perfectly rational, it might lose its human touch, making it less effective in understanding real-world complexities. In trying to iron out these flaws, we’d risk creating a robot that’s smart but clueless (think Marvin in Hitchhikers guide to the galaxy) —great with numbers but missing the point, and that may be a bigger problem than the occasional logical slip.
Implications for auditors
The reality that AI can inherit human-like biases means auditors can’t afford to trust AI outputs blindly. Instead, they must actively engage with AI’s reasoning processes, question its conclusions, and cross-check against independent, unbiased data sources. AI’s value in auditing lies in its speed and pattern recognition, but human oversight remains crucial to catch errors that are as much about psychology as they are about data.
The real solution to AI’s Linda Problem may not be just tweaking algorithms—it starts with us. Before we can teach AI not to fall for these cognitive traps, we need to understand our own minds and the flaws in our thinking. By getting a grip on why we make these mistakes, we can better train AI to avoid them, guiding it with clearer rules and smarter data. For example, by tagging on the then end of the above prompt the following line: ‘avoid the conjunction fallacy.’, the AI knows to not fall into the logic traps and instead assigns 35% to the ‘community organiser’ and a much more sensible 15% for ‘community organiser and an active member of a local environmental advocacy group’.
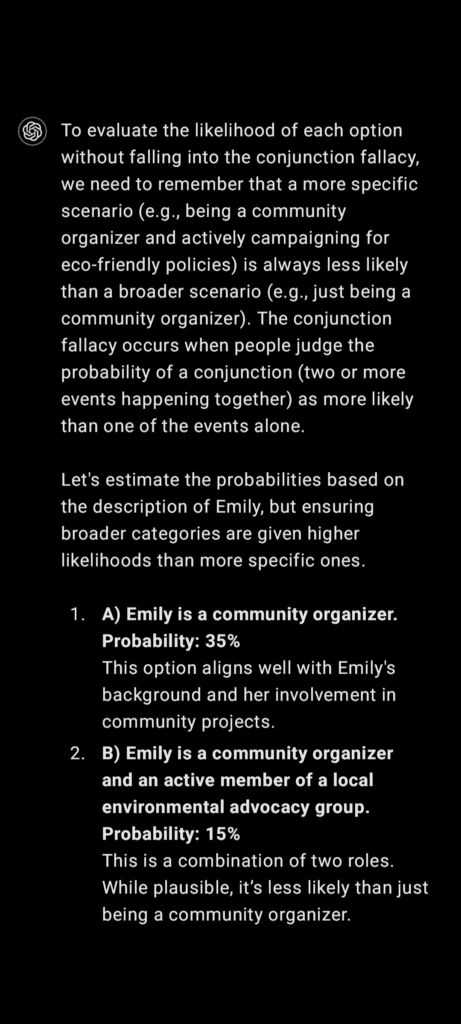
Balancing AI’s Flaws and Strengths
The idea of AI falling into the same cognitive traps as humans might seem unsettling, but it also serves as a reminder: technology, no matter how advanced, is only as good as the data and design principles that underpin it. For auditors, this means embracing AI as a tool—one that needs constant tuning and human judgment to avoid the same pitfalls we’ve been stumbling over for centuries. The future of auditing isn’t just about machines; it’s about smarter, more aware collaboration between humans and AI, ensuring we don’t just repeat the same old mistakes.